BENEFRI Summer School 2021
Organization
The workshop is organized by the University of Neuchâtel (Prof. Dr. Pascal Felber)
When
The summer school will take place in the city of Leissigen from Monday, 23 August 2021 (noon) to Wednesday, 25 August 2021 (noon).
Where
Meielisalp Hotel, Stoffelberg, 3706 Leissigen
Link to Hotel website: https://www.meielisalp.ch/de/
How to get there?
With public transportation: Get to Leissigen by train (you will have to change in Spiez). From Leissigen train station, you can take the free shuttle from the train station to the hotel (offered by the hotel, around a ten-minute ride), or walk to the hotel (43 minutes).
The shuttle will leave from the Leissigen train station at 13.00 and can carry up to 7 people per ride. If more than 7 people need the service, there will be multiple rides. To be at the meeting point on time, there is a train arriving in Leissigen at 12.51. If you are not planning to use the shuttle to reach the hotel, please let me (Antonio) know.
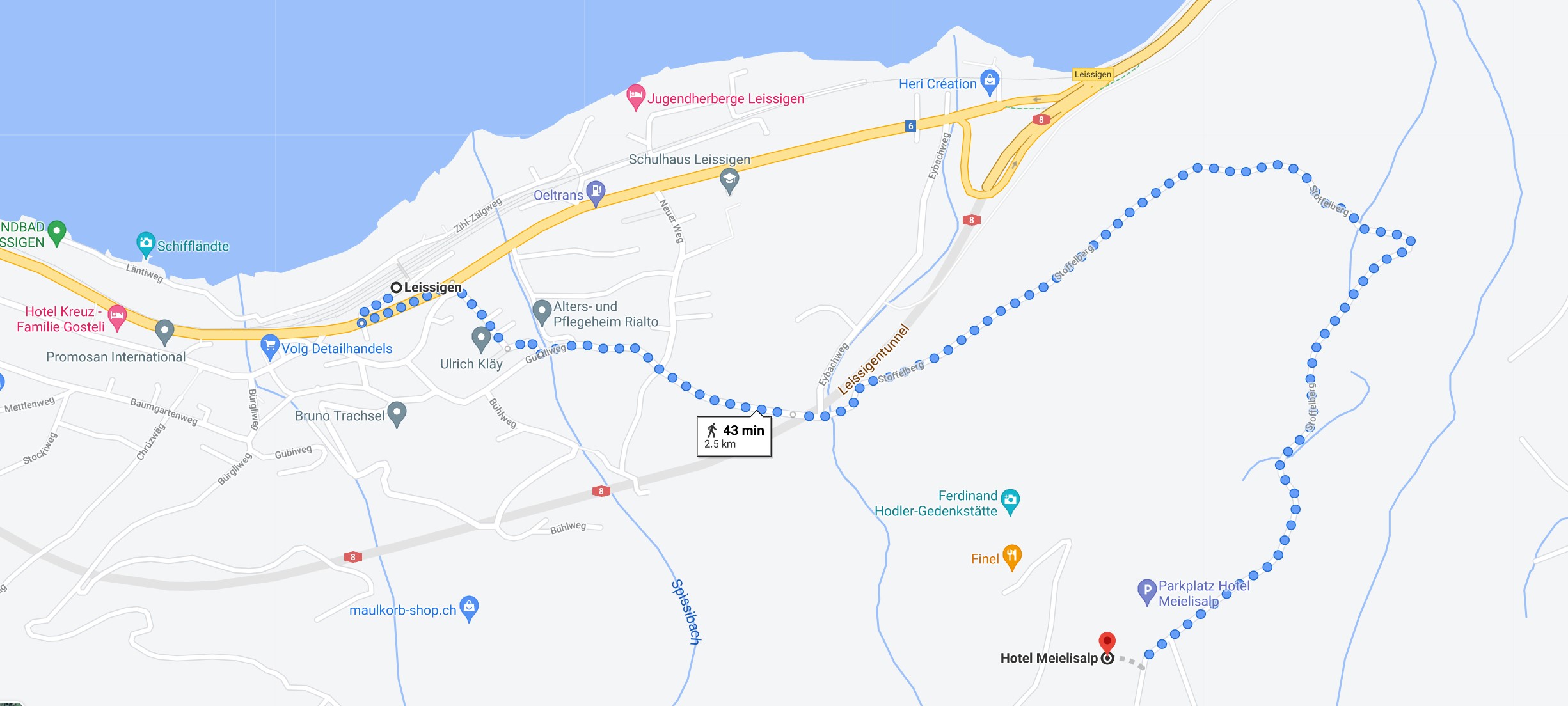
Participants List (28 people)
Students Bern (7): Negar Emami, Lucas Pacheco, Dimitris Xenakis, Diego Oliveira, Eric Samikwa, Tofunmi Ajayi, Alisson Medeiros
Students Fribourg (3): Yann Maret, Linda Studer, Frédéric Montet
Students Neuchâtel (6): Christian Göttel, Panagiotis Gkikopoulos, Rémi, Dulong, Sébastien Vaucher, Peterson Yuhala, Isabelly Rocha
Senior Bern (2): Torsten Braun, Antonio Di Maio
Senior Fribourg (5): Jean-Frédéric Wagen, Sébastien Rumley, Jean Hennebert, Pierre Kuonen, Oussama Zayene
Senior Neuchâtel (5): Pascal Felber, Peter Kropf, Valerio Schiavoni, Marcelo Pasin, Joseph Spillner,
Notes for speakers
Each talk will be 15 minutes long, followed by a 10-minute Q&A session.
Please spontaneously organize to share the same laptop among all presenters of a session to minimize the context switch penalty.
Deadline for sending your talk's title and abstract via email : Thursday, 12 August 2021
Program
Monday, 23 August 2021
Time | Content |
---|---|
14.00-14.15 | --- Welcome --- |
14.15-15.15 | Session 1: Machine Learning for Wireless Networks (chair: Antonio Di Maio) |
14.15 | Eric Samikwa (unibe) - Efficient Distributed Machine Learning for Internet of Things |
14.45 | Lucas Pacheco (unibe) - Asynchronous Federated Learning Aggregation for Vehicular Networks |
15.15 - 16.00 | --- Coffee break (included) --- |
16.00 - 17.30 | Session 2: Hardware System and Persistent Memory (chair: Sébastien Rumley) |
16.00 | Rémi Dulong (unine) - How compatible are RDMA and NVMM technologies? |
16.30 | Sebastien Vaucher (unine) - RDMA: Better, Faster, Stronger |
17.00 | Peterson Yuhala (unine) - Intel SGX Shielding for GraalVM Native Images |
19.00 | --- Dinner (included, except alcoholic beverages, in the hotel's restaurant) --- |
Tuesday, 24 August 2021
Time | Content |
---|---|
7.00-8.30 | --- Breakfast (included) --- |
9.00-10.30 | Session 3: Networked Systems I (chair: Pascal Felber) |
9.00 | Panagiotis Gkikopoulos (unine) - Leveraging Decentralized Data Quality Control to Track Quality Issues in Microservices |
9.30 | Alisson Medeiros (unibe) - Managing Edge-enabled Mobile Virtual Reality Services with Service Chaining Graph |
10.00 | Tofunmi Ajayi (unibe) - Towards A Dynamic Slicing Framework for Fog-hosted Services |
10.30-11.00 | --- Coffee break (included) --- |
11.00-12.00 | Session 4: Networked Systems II (chair: Josef Spillner) |
11.00 | Isabelly Rocha (unine) - EdgeTune: Inference Aware Multi-Parameter Tuning |
11.30 | Diego Oliveira (unibe) - Towards SDN-enabled RACH-less Make-before-break Handover in C-V2X Scenarios |
12.00 | Dimitrios Xenakis (unibe) - ARLCL: Anchor-free Ranging-Likelihood-based Cooperative Localization |
12.30-19.00 | --- Lunch (not included, lunch bags provided by the hotel for CHF 9.50) and social activity: Hike on the Lake Thun or sport activities --- |
19.00 | --- Outdoor Barbecue (included, except alcoholic beverages, at the hotel) --- |
Wednesday, 25 August 2021
Time | Content |
---|---|
7.00-8.30 | --- Breakfast (included) and check-out at 10 am --- |
9.00-10.30 | Session 5: Data Analytics (chair: Jean Hennebert) |
9.00 | Linda Studer (unifr) - Learning more from graphs |
9.30 | Negar Emami (unibe) - Artificial Neural Network-based Trajectory Prediction |
10.00-10.30 | --- Coffee break (included) --- |
10.30-12.00 | Session 6: IoT (chair: Torsten Braun) |
10.30 | Frédéric Montet (unifr) - Prediction of Domestic Hot Water Temperature in a District Heating Network |
11.00 | Christian Göttel (unine) - Scalable and Energy Efficient Trusted IoT Applications |
11.30 | Yann Maret (unifr) - Preliminary results of OLSR based MANET routing algorithms: OLSRd2-Qx reinforcement learning agents and ODRb |
12.00 | --- Farewell and final remarks --- |
Presentations Abstracts
----------
Alisson Medeiros: "Managing Edge-enabled Mobile Virtual Reality Services with Service Chaining Graph"
Abstract: "Virtual Reality (VR) enhances our physical environment by artificially rendering a real environment using audio and visual features possibly supplemented with other sensory devices. Although VR systems have attracted considerable attention in recent years, it has been considered a killer use case of the 5G landscape due to its stringent requirements. Despite expectations and investments, the use of tethered VR Head Mounted Displays (HMDs) imposes significant restrictions on VR technology’s application domain, e.g., Quality of Experience (QoE) for VR users. A primary latency bottleneck lies in the fact that VR systems are composed of multiple compute-intensive components. Furthermore, technical challenges for Mobile Virtual Reality (MVR) are posed by standalone VR HMDs that must be ergonomic, e.g., lightweight, leading to new challenges to meet the computing latency requirements for MVR merely by standalone VR HMDs processing. VR has posed several challenges to the current VR HMDs technology domain and the network infrastructure in supporting ultra-high throughput and ultra-low latency due to: (i) The current VR HMDs fail to satisfy the computing latency requirements for MVR applications; (ii) Today's VR HMDs do not support the necessary power demands; and (iii) The cloud computing architecture does not support the network latency requirements for ultimate VR applications. These challenges will become dramatically difficult to face once VR applications become advanced and massively consumed. One way to overcome the challenges mentioned above is to use the MEC infrastructure to deploy the compute-intensive tasks of VR applications. We propose refactoring VR-intensive computing tasks into VR service functions, where they are chained and deployed throughout the MEC infrastructure. We aim to reduce the computing latency for future MVR applications. However, coordinating such a plethora of service functions brings to light several challenges: (i) How would VR refactoring overcome the computational power required by VR HMDs? (ii) What are the benefits of MEC to support VR-intensive computing tasks? (iii) How to manage several VR service functions, each featuring distinct policies and requirements? To answer these questions, we propose refactoring VR services into Service Chaining Graph (SCG). SCG is a graph-based structure to support the refactoring of VR services. SCG seeks to support VR services' offloading as much as needed from the VR HMDs to the edge infrastructure."
----------
Diego Oliveira: "Towards SDN-enabled RACH-less Make-before-break Handover in C-V2X Scenarios"
Abstract: Future vehicular applications will rely on communication between vehicles and other devices in their vicinity. Technologies, such as LTE-V2X, are awaited to operate under the Cellular Vehicle-to-Everything (C-V2X) standard to make this communication possible. However, current LTE technology has to go through transformations to enhance its performance in vehicular communications. One possible enhancement for LTE is the usage of the latest handover schemes, such as RACH-less and Make-before-break (MBB), to create seamless mobility. In the presentation, we discuss a RACH-less MBB handover scheme using Software-Defined Networks (SDN) proposed by us. Our main contributions are: (i) unifying lower layer handover operations with controller network updating procedures; and (ii) creating a signaling protocol that allows base stations and controllers to exchange information needed for timing alignment of the UE without executing a RACH procedure. Simulation results show that our proposed handover scheme has a shorter execution time and reasonable signaling overhead when compared to baseline schemes from the literature.
----------
Lucas Pacheco: "Asynchronous Federated Learning Aggregation for Vehicular Networks"
Abstract: The increasing importance of robust machine learning models for network management and operation requires the massive amounts of data generated at the edge of the network for ML models training.
However, privacy and communications limitations heavily limit the amount of data learned in a centralized manner.
In this context, vehicular networks will significantly impact users' lives, as vehicles have increasing communication, storage, and computing capabilities for service consumption and driving assistance.
Federated Vehicular Networks (FVN) enable the distributed learning and processing of the data generated in modern vehicular networks by users and various sensors present in vehicles.
We present how tasks such as Federated Learning (FL) and Federated Clustering will impact modern networks and how such paradigms can be achieved in a privacy-respecting, and communication- and computationally efficient manner.
----------
Eric Samikwa: "Efficient Distributed Machine Learning for Internet of Things"
Abstract: Machine Learning (ML) is an essential technology used in (Internet of Things) IoT applications, allowing them to infer higher-level information from a large amount of raw data collected by IoT devices. However, current state-of-the-art ML models often have significant demands on memory, computation, and energy. This contradicts the resource-constrained nature of IoT devices that is characterized by limited energy budget, memory, and computation capability. Typically, ML models are trained and executed on the cloud which requires data from IoT systems to be sent to the cloud across networks for processing. Using the cloud-native approach is computationally scalable as more resources for complex analytics would be readily available, but is principally disadvantaged in various ways. Firstly, the response time obtained from processing on geographically distant data centers may not be sufficient to meet the real-time requirements of latency-critical applications. Secondly, the cloud-centric methods risk potentially exposing private and sensitive information during data transmission and remote processing and storage. Thirdly, transferring the raw data to the centralized. An alternative approach is to execute the entire tasks on end devices to mitigate the above drawbacks. However, this would require that computationally intensive models be executed on devices that are heavily constrained on computation capabilities, a challenging problem that has not been adequately addressed. To overcome the aforementioned limitations, recent studies have been proposed to make use of computing resources that are closer to data collection through distributed computing. We present a method for adaptive distribution of ML tasks in IoT networks through model partitioning for low latency and energy-efficient inference. By considering time-varying network conditions, this solution determines the optimal distribution of the task between the device and edge by selecting an ideal model partition point.
----------
Dimitris Xenakis: "ARLCL: Anchor-free Ranging-Likelihood-based Cooperative Localization"
Abstract: Different location-based services come with different positioning accuracy requirements. For outdoor applications (e.g. car navigation), most often, the global navigation satellite systems (e.g. GPS) can inexpensively cover the needs. Yet, applications in indoor environments (e.g. COVID19 tracking indoors) where the satellite signals are not available, are more challenging. Therefore, considering (i.e. fusing) many types of signal sources such as Bluetooth/WiFi signals, magnetometer, accelerometer, gyroscope, etc. is critical for achieving accurate positioning indoors. In this presentation, we propose a new methodology for enhancing the positioning accuracy in such systems by taking advantage of signals (e.g. Low Energy Bluetooth) which can be exchanged between different mobile devices (e.g. smartphones). This way, instead of tracking individually each mobile device, we consider all their exchanged signals, eventually positioning them as a system (or a swarm of devices).
----------
Negar Emami: "Artificial Neural Network-based Trajectory Prediction"
Abstract: With the advent of 5G/B5G cellular networks, mobility prediction has become crucial to enabling a wide range of network technologies and intelligent transportation services.
We present a trajectory predictor based on Convolutional Neural Networks (CNNs), whose neural architecture is designed and optimized through Reinforcement Learning (RL). RL-CNN can provide a fair compromise between prediction accuracy and training time. To manage the computational resource consumption in large-scale network scenarios, we detect similar trajectory users, build a single RL-CNN per cluster based on a few users' data, and transfer the pre-trained neural network knowledge between group members. We can save up to 90% of computational resources with this approach while losing a few percentages of the average accuracy.
----------
Peterson Yuhala: "Intel SGX Shielding for GraalVM Native Images"
Abstract: The popularity of the Java programming language has led to its wide adoption in cloud computing infrastructure. However, Java applications running in untrusted clouds are vulnerable to various forms of privileged attacks. The emergence of trusted execution environments (TEEs) such as Intel SGX mitigates this problem. TEEs protect code and data in secure enclaves inaccessible to untrusted software, including the kernel and hypervisors. To efficiently use TEEs, developers must manually partition their applications into trusted and untrusted parts, in order to reduce the trusted computing base (TCB), and minimize risks of security vulnerabilities. However, partitioning applications poses two important challenges: i) ensuring efficient object communication between the partitioned components, and ii) ensuring garbage collection consistency between the partitions, especially in memory-managed languages like Java. This work presents a tool which provides a practical and intuitive annotation-based partitioning approach for Java applications destined for secure enclaves. Our tool uses an RMI-like mechanism to ensure inter-object communication, as well as consistent garbage collection across the partitioned components.
----------
Jesutofunmi Ajayi: "Towards an Adaptive Slicing Framework for Fog Services"
Abstract: "Through network function virtualization (NFV) and Software-Defined Networking (SDN), Next-Generation Mobile Networks (i.e. 5G) are expected to support multiple services over the same physical infrastructure through the Network Slicing (NS) concept. However, ensuring that these slices (or service instances) can co-exist and operate correctly over the infrastructure will require more adaptive resource allocation approaches, that can consider the current state of resources, quality of service requirements and channel conditions, in the resource allocation and management process. Towards this, we propose an adaptive resource allocation framework, that seeks to dynamically & efficiently allocate computational resources for Fog Services in order to effectively meet the overall Quality of Service (QoS) requirements of differentiated services. We present our envisioned architecture and our current progress towards realizing such a framework, and also include some preliminary results based on a 5G NSA test-bed we have deployed."
----------
Remi Dulong: "How compatible are RDMA and NVMM technologies?"
Abstract: NVMM is a new technology in the server hardware landscape. Commercialized by Intel in 2019, its use cases are not well defined yet, as NVMM are potentially very useful to many software. This new kind of memory can be used as regular RAM memory, but its non-volatile capability is a real new-coming that requires software innovations to exploit its potential. This presentation is an overview of the technical challenges in order to use NVMM with another emerging technology: RDMA networking. This technology allows writing in another machine's RAM through the network. We think NVMM and RDMA could be used to provide new possibilities to software developers.
----------
Sebastien Vaucher: "RDMA: Better, Faster, Stronger"
Abstract: Remote direct memory access (RDMA) allows a network card to directly access the memory of another computer. Recently, RDMA has been adapted to also operate on 3D XPoint non-volatile main memory (NVMM). In this work, we use the Intel Tofino programmable switch to transparently turn RoCE—a widely-used RDMA protocol—to be point-to-multipoint. We program the switch to perform in-network data striping and mirroring, hence making NVMM over RDMA better, faster, stronger.
----------
Linda Studer: "Learning more from graphs”.
Abstract: Graph Neural Networks (GNNs) have become very popular in recent years. These frameworks extend the notion of convolution to also work with non-Euclidean data such as graphs. Graph structures have a very rich representational power, but the structure is very specific to the data at hand. Pre-training on datasets from other domains (e.g. ImageNet), which is common for Convolutional Neural Networks (CNNs) is not feasible for graphs, as the graph representations are very closely tied to the data type, i.e., the node and graph labels, and the meaning of the edges. Therefore, we are investigating how to enrich the existing graph structures by adding a central node and a complimentary graph. I will present our preliminary results obtained on synthetic handwritten data. Furthermore, this synthetic data can also be used to pre-train GNNs for real-world tasks involving handwritten data.
----------
Christian Göttel: "Scalable and Energy Efficient Trusted IoT Applications"
Abstract: In this talk, I will present a summary of my thesis on trusted IoT applications. The first part briefly describes Internet of things (IoT) and presents the Raspberry Pi as an IoT prototyping platform. Afterwards, trusted execution environments (TEE) are introduced, namely, ARM TrustZone, Intel SGX, and AMD SEV, which shield trusted applications from malicious users and compromised systems. We then compare the performance and scalability before following up with a discussion on the advantages, drawbacks, and compromises of these TEEs for different kinds of use cases. For the second part, we begin with an introduction to energy efficiency, the different units that have been proposed, and their pitfalls. We then look again at the use cases presented in the first part and compare the energy efficiency for the different TEEs. To conclude my talk, I will exhibit a novel attack scenario called scrooge attack. This recently published research assumes an undervolted cloud infrastructure and demonstrates how cloud users can detect such infrastructure under a very strong threat model.
----------
Yann Maret: Preliminary results of OLSR based MANET routing algorithms: OLSRd2-Qx reinforcement learning agents and ODRb
Abstract: In MANETs, congestion typically occurs on the interconnecting nodes between two or more groups of nodes. Routing to avoid the congested nodes via alternate, perhaps longer paths, allows more throughput, e.g., 50% more in the canonical 9-node 2-ring scenario. OLSR-Q is based on the routing protocol OLSR and a reinforcement learning (RL) agent to learn the most appropriate link states or “Directional Air Time” metric to avoid the congested nodes. The challenges for the RL agent are (1) to avoid congestion before packets are dropped and (2) to minimize the number of real valued or discrete observations or states. In this paper, three simplified OLSRd2-Qx versions are presented and compared to OLSRd2 and a centralized ODRb, Omniscient Dijkstra Routing-balanced, algorithm. The proposed OLSRd2-Qload algorithm provides the expected 50% increase in throughput on the 9-node 2-ring scenario with a specific test traffic scenario. On the NATO IST-124 Anglova scenario, and using an acknowledged message application, the Q-learning agents remain to be improved. The superior results of the centralized load balancing approach taken in ODRb will be investigated to train multi-agents systems including OLSR-Q.
----------
Panagiotis Gkikopoulos: "Leveraging Decentralized Data Quality Control to Track Quality Issues in Microservices"
Abstract: Autonomic, data-driven systems that automate decision making based on monitoring and data capture are becoming more common in practice. However, acquiring the data to power these systems and ensuring their quality is an ongoing challenge. System failures and software errors can cause irreversible data loss or corruption, thus reducing the reliability of the system that relies on said data. We propose a decentralized and collaborative approach to data management, that relies on reproducible data acquisition and group-consensus QA automation for the establishment of ground truth among participants and the improvement of data quality. We present different strategies to achieve this dynamic data enhancement and evaluate our method’s effects on the data and their performance. Finally, we study the application of this methodology on tracking the evolution of quality issues in cloud-native software artifacts.
----------
Frédéric Montet: "Prediction of Domestic Hot Water Temperature in a District Heating Network"
Abstract: With the fourth generation of district heating networks in sight, opportunities are rising for better services and optimized planning of energy production. Indeed, the more intensive data collection is expected to allow for load prediction, customer profiling, etc. In this context, our work aims at a better understanding of customer profiles from the captured data. Given the variety of households, such profiles are difficult to capture. This study explores the possibility to predict domestic hot water (DHW) usage. Such prediction is made challenging due to the presence of two components in the signal, the first one bound to the physical properties of the DHW distribution system, the second one bound to the human patterns related to DHW consumption. Our contributions include (1) the analysis of recurrent neural network architectures based on GRU, (2) the inclusion of state-based labels inferred in an unsupervised way to simulate domain knowledge, (3) the comparison of different features. Results show that the physical contribution in the signal can be forecasted successfully across households. On the contrary, the stochastic ”human” component is harder to predict and would need further research, either by improving the modeling or by including alternate signals.
----------
Isabelly Rocha: "EdgeTune: Inference Aware Multi-Parameter Tuning"
Abstract: Deep Neural Networks (DNNs) have demonstrated impressive performance on many machine-learning tasks such as image recognition and language modeling, and are becoming prevalent even on mobile platforms. Despite so, designing neural architectures still remains a manual, time-consuming process that requires profound domain knowledge. Recently, Parameter Tuning Servers have gathered the attention o industry and academia. Those systems allow users from all domains to automatically achieve the desired model accuracy for their applications. However, although the entire process of tuning and training models is performed solely to be deployed for inference, state-of-the-art approaches typically ignore system-oriented and inference-related objectives such as runtime, memory usage, and power consumption. This is a challenging problem: besides adding one more dimension to an already complex problem, the information about edge devices available to the user is rarely known or complete. To accommodate all these objectives together, it is crucial for tuning systems to take a holistic approach to parameter tuning and consider all levels of parameters simultaneously into account. We present EdgeTune, a novel inference-aware parameter tuning server. It considers the tuning of parameters in all levels backed by an optimization function capturing multiple objectives. Our approach relies on inference estimated metrics collected from our emulation server running asynchronously from the main tuning process. The latter can then leverage the inference performance while still tuning the model. We propose a novel one-fold tuning algorithm that employs the principle of multi-fidelity and simultaneously explores multiple tuning budgets, which the prior art can only handle as suboptimal case of single type of budget. EdgeTune outputs inference recommendations to the user while improving tuning time and energy by at least 18% and 53% when compared to the baseline Tune.
----------